Is Data Science Worth It? (Or is ChatGPT king?!)
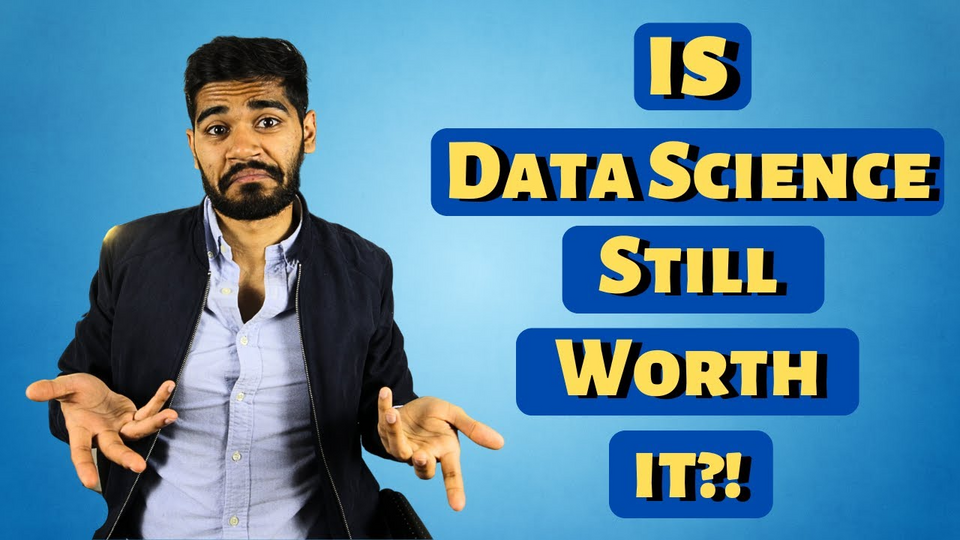
As the field of Artificial Intelligence (AI) continues to evolve, questions arise about the future of data science. With the emergence of Large Language Models (LLMs), such as ChatGPT and leaked Facebook model LLaMA, there's been a significant shift in the AI community's focus. In this article, we will discuss whether data science is still worth pursuing amidst the rising popularity of LLMs. I'm Samanvay, a data scientist based in Sydney, Australia, and I'll shed light on this intriguing topic.
The Rise of Large Language Models
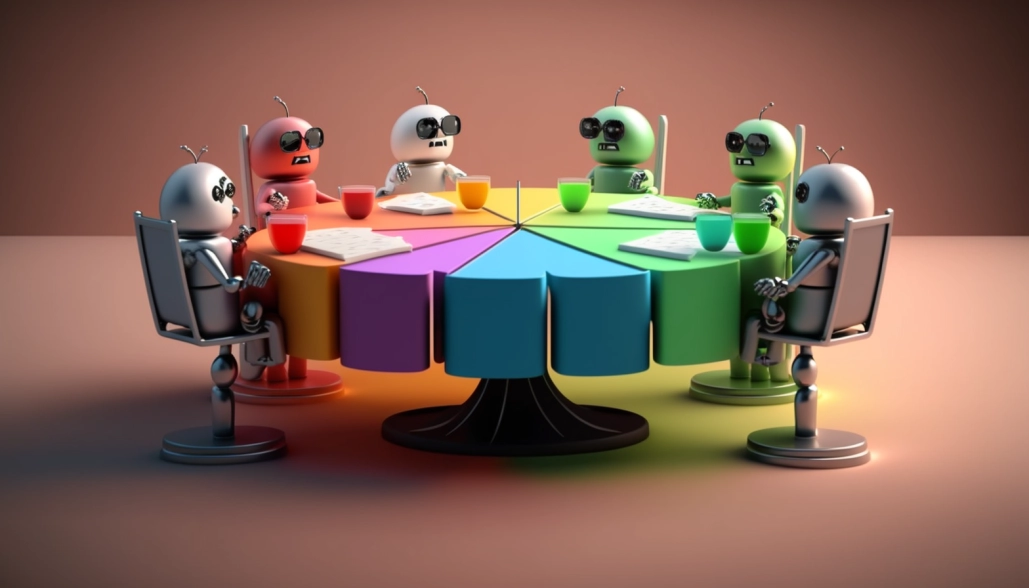
The AI landscape has been abuzz with excitement over LLMs since the release of ChatGPT and LLaMA. These models have gained popularity, sparking conversations within the AI community and even prompting companies to develop their own LLMs. LinkedIn has witnessed a surge of self-proclaimed AI experts overnight. This new wave of AI has led me to question the worth of pursuing data science in recent times. It seems that AI is becoming increasingly commoditised, with companies adopting AI as a service.
The Changing Role of Data Scientists
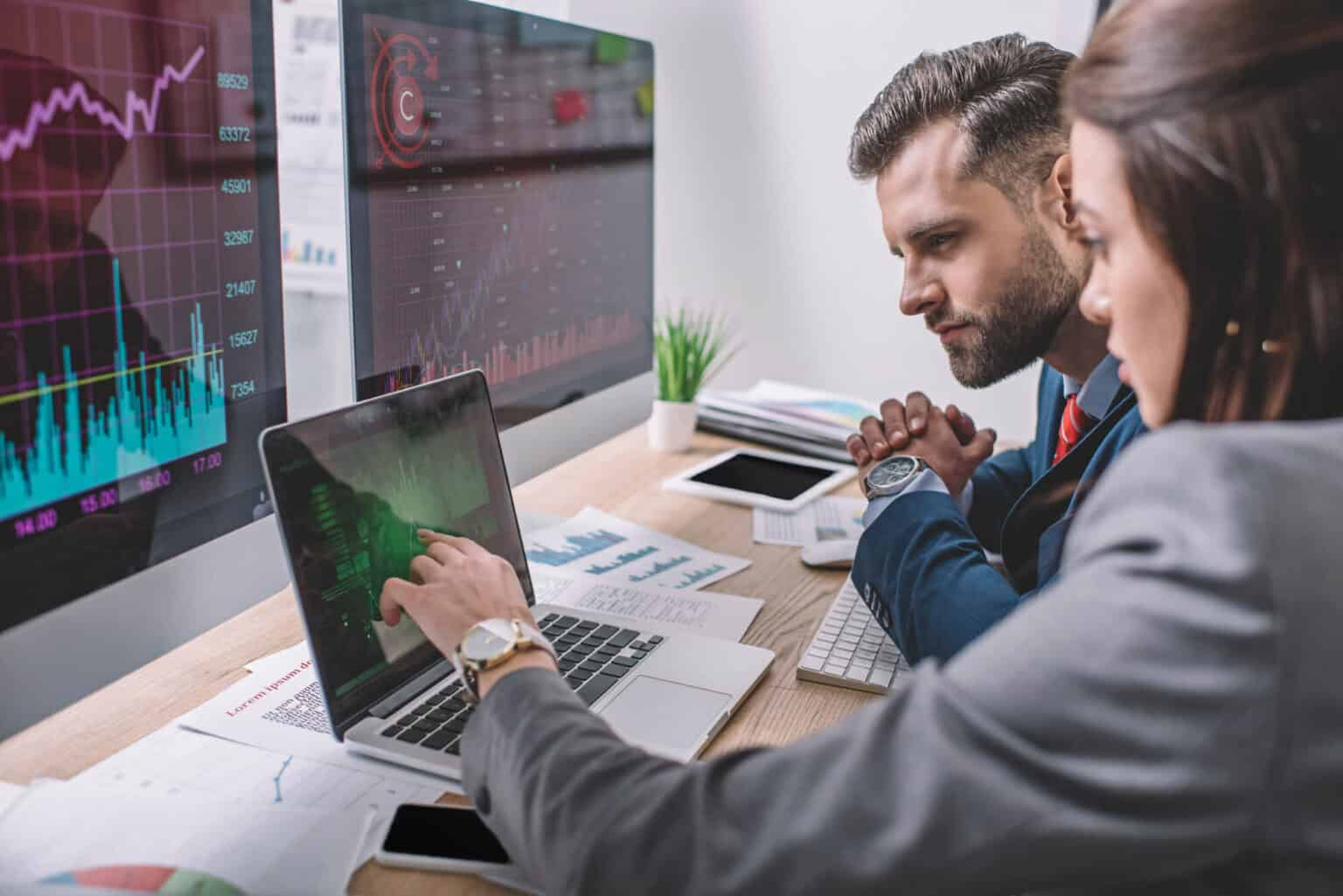
While AI is being embraced as a feature in various products, the role of the data scientist is not obsolete. However, it is evolving. Modern data scientists are now responsible for creating innovative data products in a world where AI is readily available. Merely becoming a proficient engineer is not the most appealing job description for a data scientist. Instead, they must possess a deep understanding of the specific domain they are working in. For example, a data scientist in the finance sector should be well-versed in financial problems rather than relying on generic models. In a world where AI is accessible as a service, data scientists must focus on delivering business outcomes and be product-focused.
Would rather watch a view? Check it out 👇
The Importance of Hands-On Skills and Soft Skills
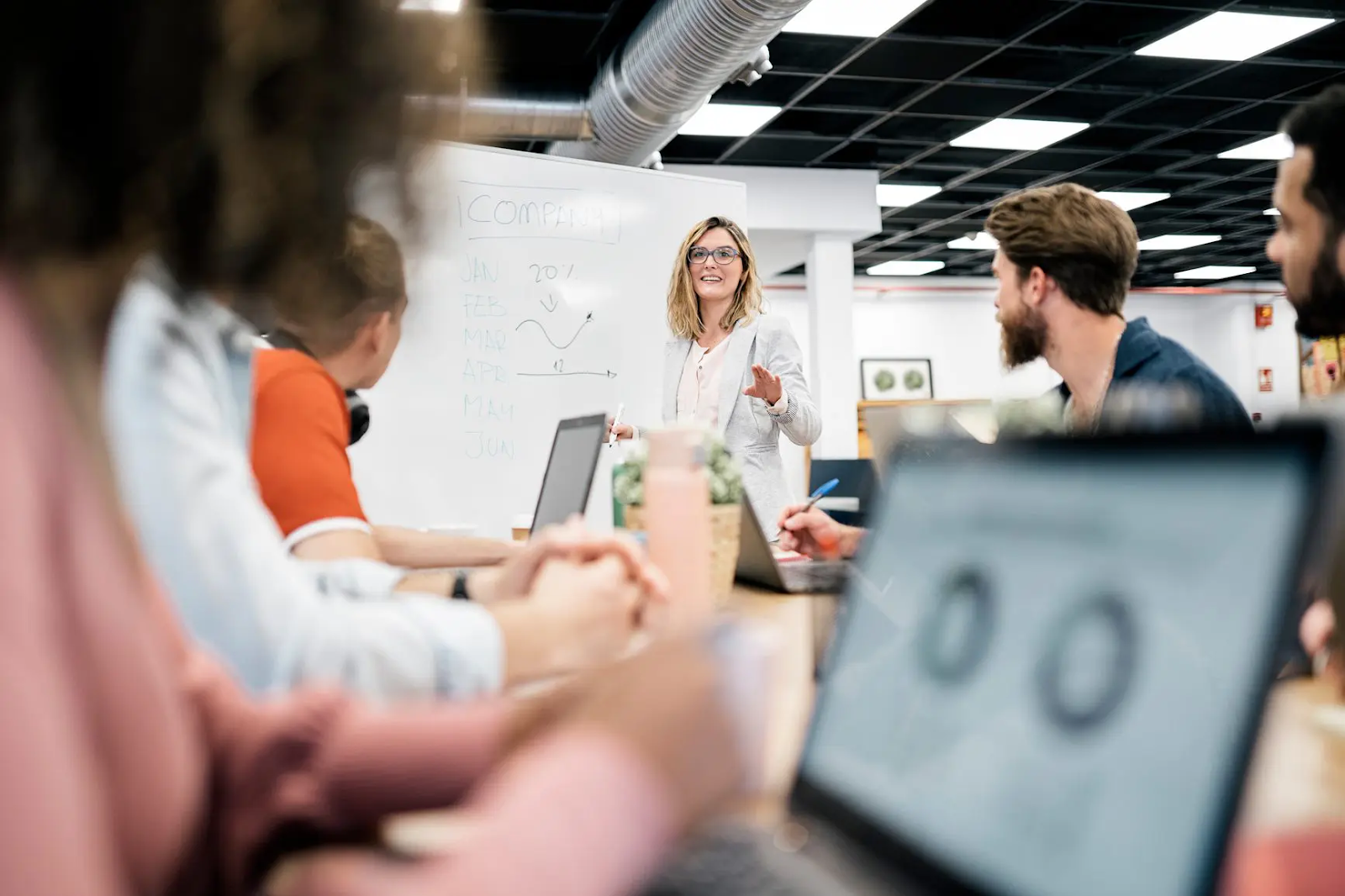
Data scientists now need to have hands-on coding skills to build data pipelines around LLMs and leverage data infrastructure effectively. Soft skills also play a crucial role as data scientists spend more time scoping problems and designing data solutions rather than simply building models. The ability to communicate effectively and collaborate with stakeholders becomes essential in this context.
The Impact of Large Language Models on Data Science Jobs
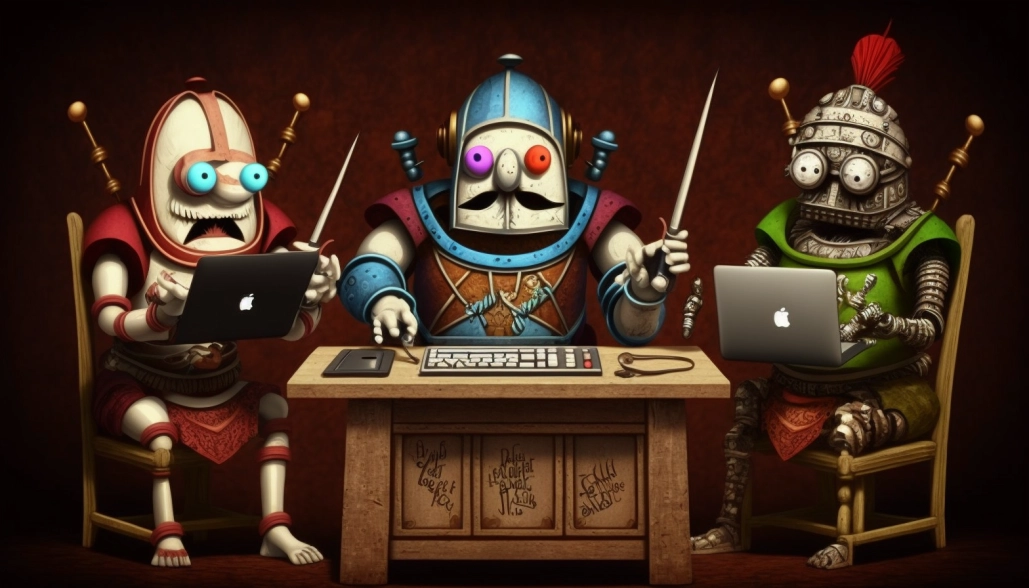
With the advancement of LLMs, the number of data scientists hired solely for building models will decrease significantly. However, even before the introduction of LLMs, there were only a few data scientists exclusively dedicated to model building. Simpler models, like tree-based models, will still be in demand. It's important to remember that not every data problem requires an LLM. Therefore, the need for skilled data scientists who can determine the right model for each specific problem remains.
The Future of Data Science
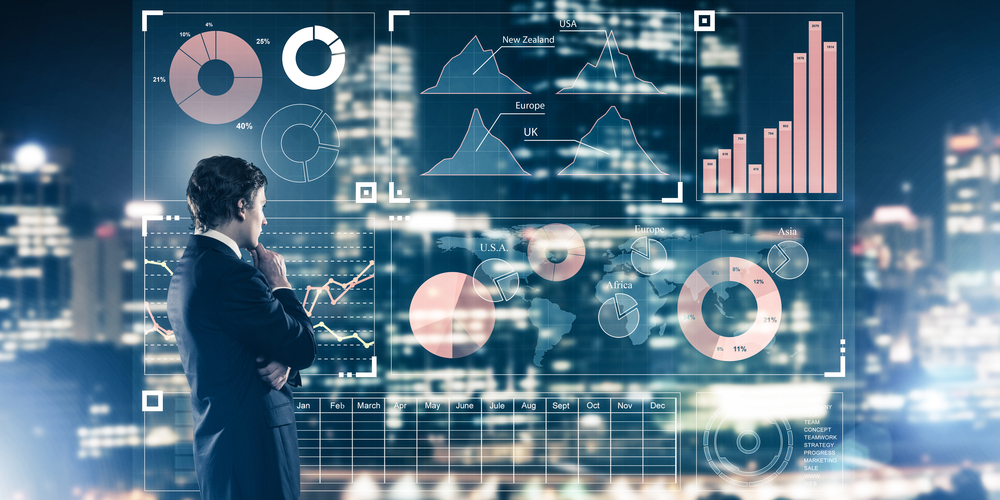
Despite the rise of LLMs, data science continues to hold value. However, data scientists must be adaptable and resilient. They need to upskill rapidly and keep pace with the fast-evolving world of AI innovation. Staying updated with open-source tools and actively practising data science is essential. Many APIs are freely available for experimentation, allowing aspiring data scientists to explore different GPT variants and create their own data products. The best way to learn data science is through hands-on experience.
Conclusion
In conclusion, data science is still worth pursuing, even in the age of LLMs. The field is evolving, and data scientists must adapt to the changing landscape. By focusing on delivering business outcomes, leveraging hands-on coding skills, and honing soft skills, data scientists can continue to thrive. The key lies in staying up-to-date with AI innovations and actively engaging with open-source tools. So, if you're passionate about data science, embrace the opportunities and start creating your own data products.
If you found this article helpful, please show your support by sharing it. Stay tuned for more valuable insights and guidance. Until next time!